Thursday, November 16th, 2023

Sam Hopkins, Massachusetts Institute of Technology
Synopsis
While statistical learning theory gives reliable foundations for estimation and inference from ideal data sets, real world data sets are quite far from the ideal. With sources of imperfections such as noisy observations, miscoded values, missing values, and distribution shift between collection and implementation time, it has now become critical to develop tools which can give robust insights from such imperfect data.

Sivaraman Balakrishnan, Carnegie Mellon University
Logistics
- Date: Thursday, November 16, 2023
- In-person Location: Northwestern University: Mudd Library 3rd floor, 2233 Tech Drive, Evanston
- Register: Click here to register
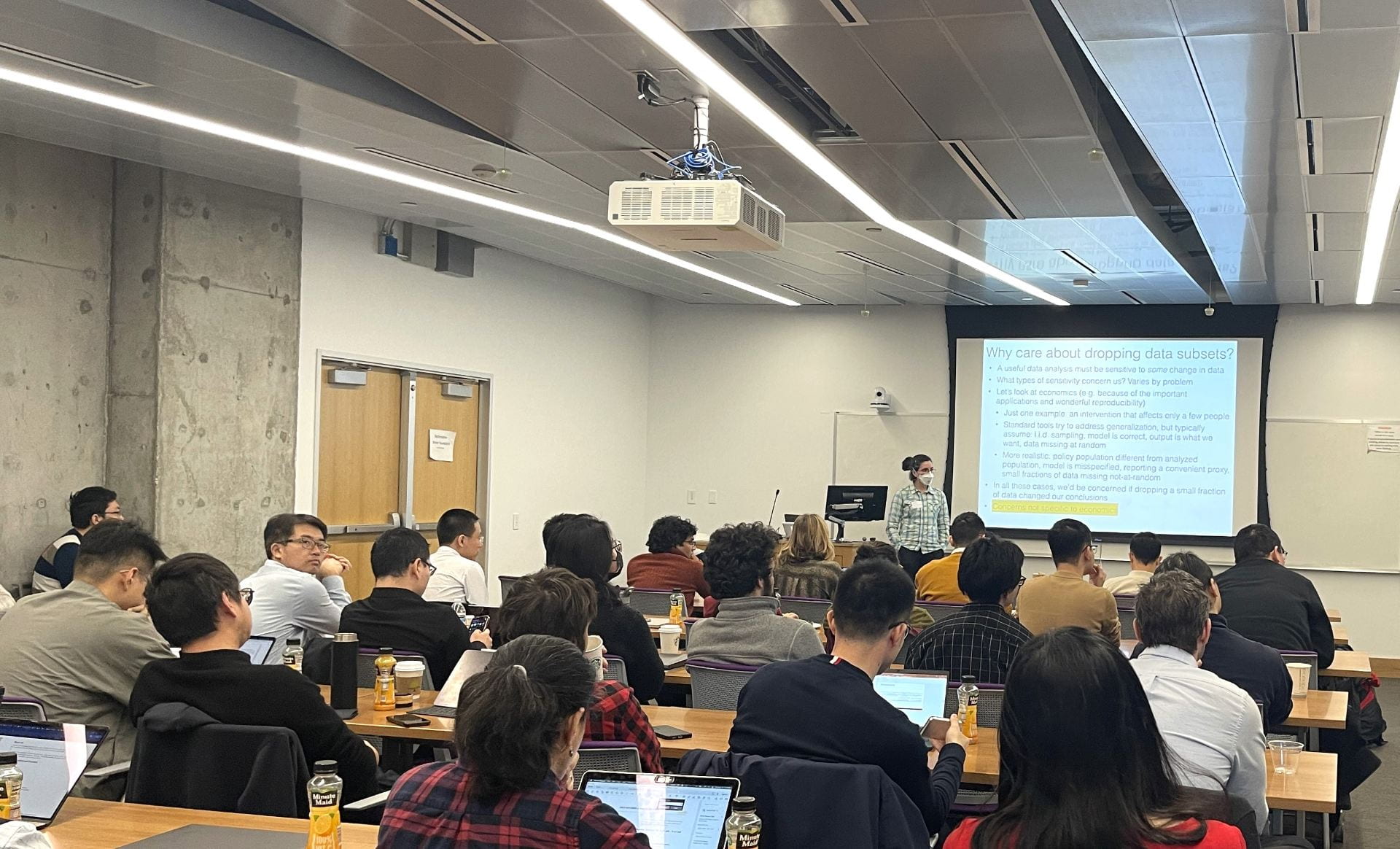
Tamara Broderick, Massachusetts Institute of Technology
Schedule (more details are forthcoming)
9:15 – 9:50 Breakfast
9:50 – 10:00 Opening remarks
10:00 – 10:45 Sam Hopkins (MIT)
10:45 – 11:30 Sivaraman Balakrishnan (CMU)
11:30 – 11:45 Coffee break
11:45 – 12:30 Tamara Broderick (MIT)
- Nian Si (University of Chicago)
- Subhodh Kotekal (University of Chicago)
- Yuzhang Shang (IIT)
5:15- Dinner
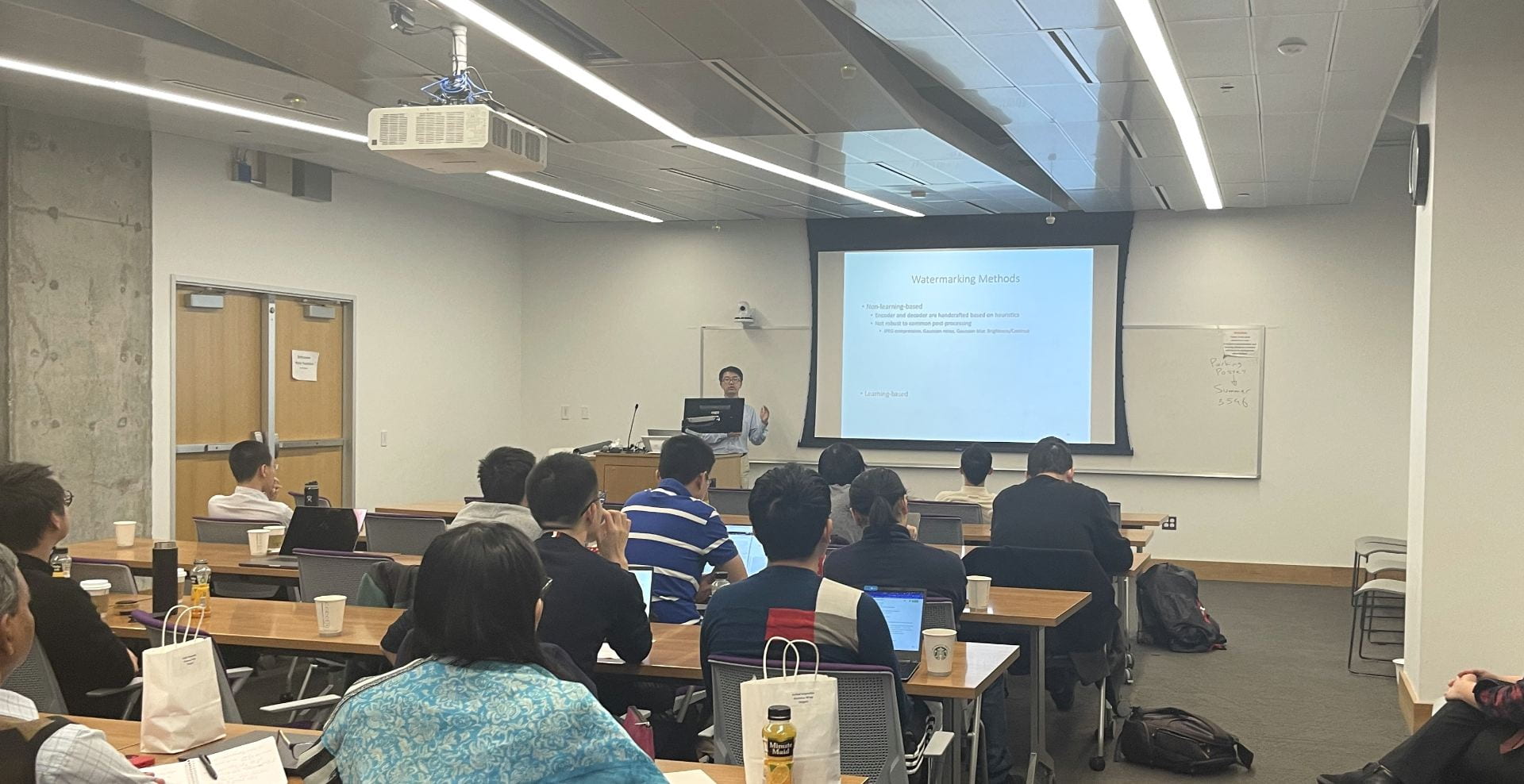
Neil Gong, Duke University
Titles and Abstracts
Speaker: Sivaraman Balakrishnan
Title: Minimax Theory for Causal Inference
Abstract: One of the most salient examples of attempting to make rigorous inferences from imperfect data is in attempting to infer causal quantities from observational data. The causal analysis of (observational) data plays a central role in essentially every scientific field. Many recent developments in causal inference, and functional estimation problems more generally, have been motivated by the fact that classical one-step de-biasing methods, or their more recent sample-split double machine-learning avatars, can outperform plugin estimators under surprisingly weak conditions. However, from a theoretical perspective our understanding of how to construct these estimators for non-standard functionals, how to assess their optimality, and how to improve them, is still far from complete.
I will present two vignettes within this theme. The first part develops minimax theory for estimating the conditional average treatment effect (CATE). Many methods for estimating CATEs have been proposed, but there remain important theoretical gaps in understanding if and when such methods are optimal. We close some of these gaps, by providing sharp minimax-rates for estimating the CATE when the nuisance functions are Holder smooth — highlighting important differences between the estimation of the CATE, and its more well-studied global counterpart (the average treatment effect).
____
Speaker: Tamara Broderick
Title: An Automatic Finite-Sample Robustness Check: Can Dropping a Little Data Change Conclusions?
Abstract: Practitioners will often analyze a data sample with the goal of applying any conclusions to a new population. For instance, if economists conclude microcredit is effective at alleviating poverty based on observed data, policymakers might decide to distribute microcredit in other locations or future years. Typically, the original data is not a perfect random sample from the population where policy is applied — but researchers might feel comfortable generalizing anyway so long as deviations from random sampling are small, and the corresponding impact on conclusions is small as well. Conversely, researchers might worry if a very small proportion of the data sample was instrumental to the original conclusion. So we propose a method to assess the sensitivity of statistical conclusions to the removal of a very small fraction of the data set. Manually checking all small data subsets is computationally infeasible, so we propose an approximation based on the classical influence function. Our method is automatically computable for common estimators. We provide finite-sample error bounds on approximation performance and a low-cost exact lower bound on sensitivity. We find that sensitivity is driven by a signal-to-noise ratio in the inference problem, does not disappear asymptotically, and is not decided by misspecification. Empirically we find that many data analyses are robust, but the conclusions of several influential economics papers can be changed by removing (much) less than 1% of the data.
____
Speaker: Neil Gong
Title: Secure Content Moderation for Generative AI
Abstract: Generative AI–such as GPT-4 and DALL-E 3–raises many ethical and legal concerns such as the generation of harmful content, scaling disinformation and misinformation campaigns, as well as disrupting education and learning. Content moderation for generative AI aims to address these ethical and legal concerns via 1) preventing a generative AI model from synthesizing harmful content, and 2) detecting AI-generated content. Prevention is often implemented using safety filters, while detection is implemented by watermark. Both prevention and watermark-based detection have been recently widely deployed by industry. In this talk, we will discuss the security of existing prevention and watermark-based detection methods in adversarial settings.
____
Speaker: Sam Hopkins
Title: The Full Landscape of Robust Mean Testing: Sharp Separations between Oblivious and Adaptive Contamination
Abstract: We consider the question of Gaussian mean testing, a fundamental task in high-dimensional distribution testing and signal processing, subject to adversarial corruptions of the samples. We focus on the relative power of different adversaries, and show that, in contrast to the common wisdom in robust statistics, there exists a strict separation between adaptive adversaries (strong contamination) and oblivious ones (weak contamination) for this task. We design both new testing algorithms and new lower bounds to show that robust testing in the presence of an oblivious adversary requires strictly fewer samples than in the presence of an adaptive one. Joint work with Clement Canonne, Jerry Li, Allen Liu, and Shyam Narayanan, appeared in FOCS 2023.
____
Speaker: Subhodh Kotekal
Title: Optimal null estimation in the two-groups model
Abstract: The advent of large-scale inference has spurred reexamination of conventional statistical thinking. In a series of highly original articles, Efron showed in some examples that the ensemble of the null distributed test statistics grossly deviated from the theoretical null distribution, and Efron persuasively illustrated the danger in assuming the theoretical null’s veracity for downstream inference. Though intimidating in other contexts, the large-scale setting is to the statistician’s benefit here. There is now potential to estimate, rather than assume, the null distribution. We adopt Efron’s suggestion and consider rate-optimal estimation of the null in the two-groups model without imposing any assumptions on the nonnull effects. The minimax upper bound is obtained by considering estimators based on the empirical characteristic function and the classical kernel mode estimator. Faster rates than those in Huber’s contamination model are achievable by exploiting the Gaussian character of the data.
____
Speaker: Nian Si (collaborative work with Shengbo Wang (Stanford), Jose Blanchet (Stanford), and Zhengyuan Zhou (NYU Stern))
Title: On the Foundation of Distributionally Robust Reinforcement Learning
Abstract: Motivated by learning a good policy that is robust to environment shifts between training time (i.e., in a simulator) and test time (i.e., in a real environment), we contribute to the theoretical foundation of distributionally robust reinforcement learning (DRRL). We accomplish this through the introduction of a comprehensive modeling framework centered around distributionally robust Markov decision processes (DRMDPs). This framework obliges the decision maker to select an optimal policy under the worst-case distributional shift provisioned by an adversary. By unifying and extending existing formulations in the literature, we provide a rigorous construction of a DRMDP, which encompasses several modeling attributes pertaining to both the decision maker and the adversary. These attributes include the granularity at which the decision maker and/or the adversary can adapt, where we examine three common scenarios including history-dependent, Markov, and Markov time-homogeneous decision maker/adversary. Furthermore, we explore the flexibility of shifts that the adversary can induce, where we further examine the SA-rectangular and S-rectangular adversaries.
____
Speaker: Victor Veitch
Title: Linear Structure of High-Level Concepts in Text-Controlled Generative Models
Abstract: Text controlled generative models (such as large language models or text-to-image diffusion models) operate by embedding natural language into a vector representation, then using this representation to sample from the model’s output space. This talk concerns how high-level semantics are encoded in the algebraic structure of representations. In particular, we look at the idea that such representations are “linear”—what this means, why such structure emerges, and how it can be used for precision understanding and control of generative models.
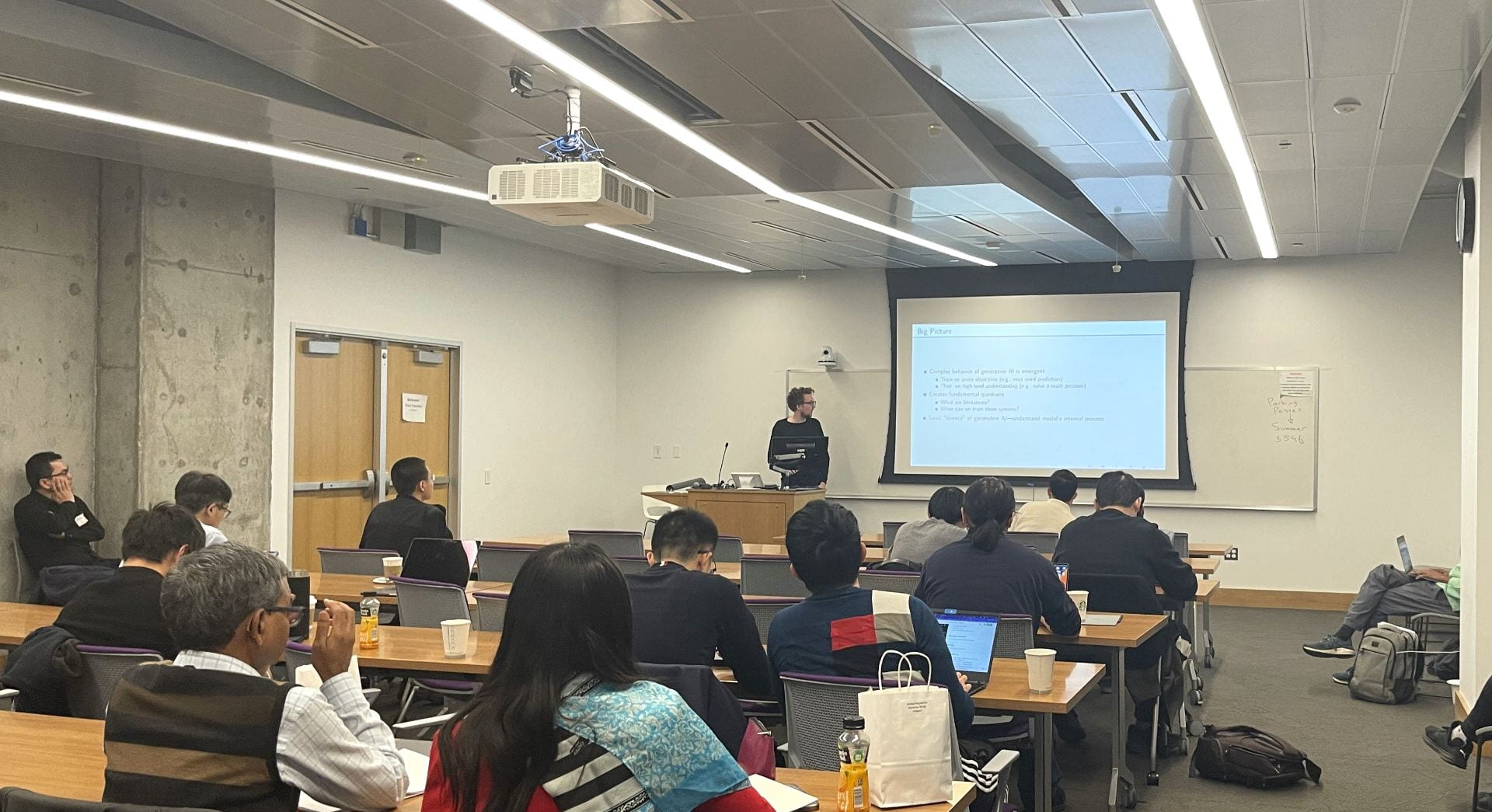
Victor Veitch, University of Chicago
Organizers
- Chao Gao (University of Chicago)
- Varun Gupta (University of Chicago)
- Binghui Wang (Illinois Institute of Technology)